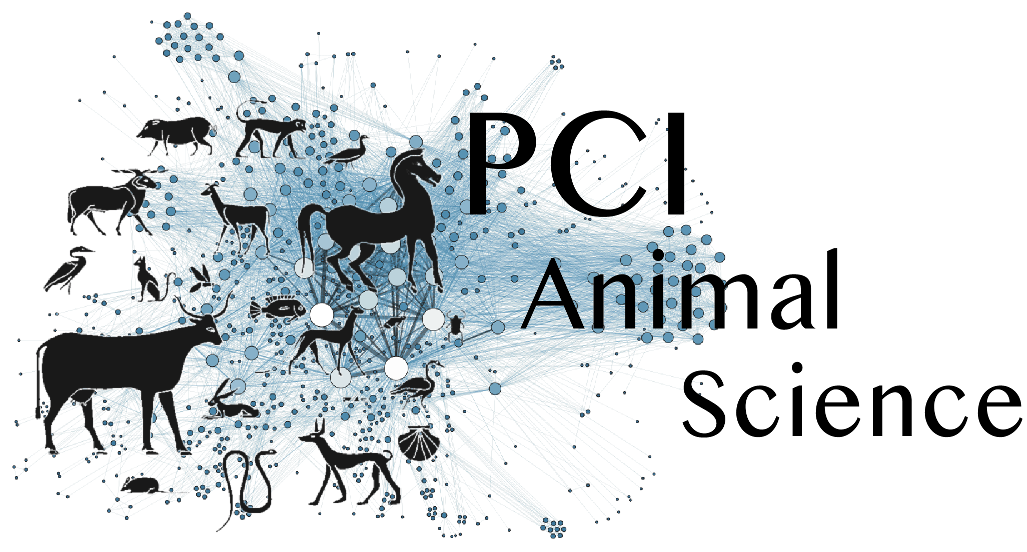
Introducing a feature extraction method and implementing a gradient boosting algorithm to predict behavioral traits in animals
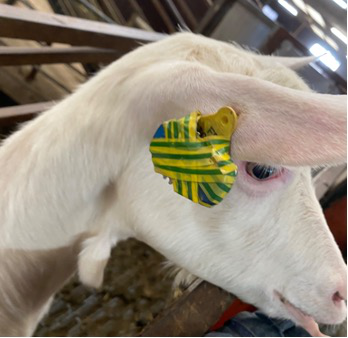
A pipeline with pre-processing options to detect behaviour from accelerometer data using Machine Learning tested on dairy goats.
Abstract
Recommendation: posted 01 April 2025, validated 03 April 2025
Rafat, S. (2025) Introducing a feature extraction method and implementing a gradient boosting algorithm to predict behavioral traits in animals. Peer Community in Animal Science, 100341. https://doi.org/10.24072/pci.animsci.100341
Recommendation
In the livestock industry, the concerns of society and livestock farmers have changed in recent decades, moving from a focus solely on production traits to sustainable production while respecting animal welfare (Ducrot et al., 2024). Animal behavior parameters can serve as a reliable proxy for animal welfare, but collecting large data sets of behavioral data on farm is very time-consuming when not automatized. Accelerometers are promising devices to detect animal behavior. A key element of the efficiency of the prediction of animal behavior from accelerometer data is the adequacy of pre-processing methods (Riaboff et al., 2019,Riaboff et al., 2022, Vidal et al., 2023).
The article of Mauny et al., (2025) aims to find a solution for using huge automatized data. So, livestock farmers can effectively use new technologies to monitor animal behavior and then correct poor husbandry routines. Authors used the pipeline ACT4Behav - (Accelerometer-based Classification Tool for identifying Behaviours) - (Mauny et al., 2024) for the pre-processing of accelerometer data with the aim of selecting the most important features of dairy goats related to rumination, head in the feeder, lying and standing (using data based on video recordings for the validation of behavior activity). Mauny et al., (2025) established a clear methodology that systematically obtain the best features and processing techniques to predict each targeted variable from raw accelerometer data. The work provides valuable information for both animal husbandry specialists and data mining scientists. The main limitation of the work is the small number of animals for both training and model testing.
References
Ducrot C, Barrio MB, Boissy A, Charrier F, Even S, Mormède P, Petit S, Pinard-van der laan M-H, Schelcher F, Casabianca F, Ducos A, Foucras G, Guatteo R, Peyraud J-L, Vayssier-Taussat M, Veysset P, Friggens NC and Fernandez X. 2024. Animal board invited review: Improving animal health and welfare in the transition of livestock farming systems: Towards social acceptability and sustainability. animal 18, 101100. https://doi.org/10.1016/j.animal.2024.101100
Mauny, S, Kwon J, Friggens NC, Duvaux-Ponter C, Taghipoor M. 2024. ACT4Behav (Accelerometer-based Classification Tool for identifying Behaviours): a Machine Learning pipeline with extensive pre-processing and feature creation options. Zenodo. https://doi.org/10.5281/zenodo.12624796
Mauny S, Kwon J, Friggens NC, Duvaux-Ponter C, Taghipoor M. 2025. A pipeline with pre-processing options to detect behaviour from accelerometer data using Machine Learning tested on dairy goats.. Zenodo, ver. 6 peer-reviewed and recommended by PCI Animal Science. https://doi.org/10.5281/zenodo.12627197
Riaboff L, Aubin S, Bédère N, Couvreur S, Madouasse A, Goumand E, Chauvin A, Plantier G. 2019. Evaluation of pre-processing methods for the prediction of cattle behaviour from accelerometer data. Computers and Electronics in Agriculture 165, 104961. https://doi.org/10.1016/j.compag.2019.104961
Riaboff L, Shalloo L, Smeaton AF, Couvreur S, Madouasse A, Keane MT. 2022. Predicting livestock behaviour using accelerometers: A systematic review of processing techniques for ruminant behaviour prediction from raw accelerometer data. Computers and Electronics in Agriculture 192, 106610. https://doi.org/10.1016/j.compag.2021.106610
Vidal G, Sharpnack J, Pinedo P, Tsai IC, Lee AR, Martínez-López B. 2023. Impact of sensor data pre-processing strategies and selection of machine learning algorithm on the prediction of metritis events in dairy cattle. Preventive Veterinary Medicine 215, 105903. https://doi.org/10.1016/j.prevetmed.2023.105903
The recommender in charge of the evaluation of the article and the reviewers declared that they have no conflict of interest (as defined in the code of conduct of PCI) with the authors or with the content of the article. The authors declared that they comply with the PCI rule of having no financial conflicts of interest in relation to the content of the article.
ANR-22-PEAE-0008
Evaluation round #4
DOI or URL of the preprint: https://doi.org/10.5281/zenodo.12627197
Version of the preprint: 4
Author's Reply, 01 Apr 2025
Dear Dr. Rafat,
Thank you for your valuable feedback.
To address this, we have included a reference in the M&M section to a study that describes the milk yield, body weight, and dry matter intake of these goats.
Best regards,
Sarah M
Decision by Seyed Abbas Rafat
, posted 28 Mar 2025, validated 28 Mar 2025
Dear authors,
Thank you for the revised manuscript.
The methodology is clearly presented, however it would be interested to readers if the conditions of the 8 goats were also provided (for example any information concerning age, parity, height, milk production, month of lactation, and body weight).
This seems to be a limitation of the paper, but it may be possible to provide this information at this round.
Best Regards,
S. A. Rafat
Evaluation round #3
DOI or URL of the preprint: https://doi.org/10.5281/zenodo.14499757
Version of the preprint: 3
Author's Reply, 25 Mar 2025
Dear Managing Board,
Thank you for your message. I have updated the manuscript to include the reference to my recently published data paper and have deposited the revised version on Zenodo.
Please let me know if you need anything else.
Best regards,
Sarah Mauny
Decision by Seyed Abbas Rafat
, posted 25 Mar 2025, validated 25 Mar 2025
Dear Sarah Mauny,
You article is about to be recommended. Can you please deposit in zenodo a new version of the manuscript, including the reference of your data paper recently published: https://doi.org/10.1016/j.anopes.2025.100095
We consider it is important to include this reference given that it explains the data you used in the manuscript submitted to PC Animal Science.
Please let us know when the new version is posted.
Thanks
Best regards
The managing board
Evaluation round #2
DOI or URL of the preprint: https://doi.org/10.5281/zenodo.14178117
Version of the preprint: 2
Author's Reply, 16 Dec 2024
We sincerely thank you for your positive feedback and for appreciating the efforts we made to address the comments from the previous round of revisions. We have carefully considered your last suggestions and made the requested editorial changes to further improve the manuscript.
We hope these changes fully address the reviewers' and editors' requests.
Thank you again for your constructive comments.
Best regards,
Mauny Sarah
On behalf of all co-authors
Decision by Kristan Reed, posted 12 Dec 2024, validated 13 Dec 2024
The authors did an excellent job responding to the reviewer's comments. I believe the alteration of the title and small additions to the methods have improved the clarity. I would like to request just a few editorial changes to correct typos and and suggest one change to the conclusion before providing my recommendation:
L238 “time-series”
L265 “Area Under the Curve” or just “AUC”
L350 missing end of parentheses… “additional time series (median…) (Table 4)
L430 “pre-process” not “pre-pre-processed”
The last sentence in the conclusion cites application of a method in a paper that is in preparation. I suggest just removing this citation as I don't think it belongs in the conclusion and the referenced work is not yet accessible anyway.
Comment from the managing board. In the Conflict of interest disclosure section, please add the following sentence: “The authors declare the following non-financial conflict of interest: Masoomeh Taghipoor and Nicolas Friggens are recommenders of PCI Animal Science”.
Evaluation round #1
DOI or URL of the preprint: https://doi.org/10.5281/zenodo.12627197
Version of the preprint: 1
Author's Reply, 18 Nov 2024
Decision by Kristan Reed, posted 04 Oct 2024, validated 05 Oct 2024
Both reviewers and myself find a lot of merit in the work presented and the inclusion of the supporting code is a particularly valuable contribution. Before recommendation, I would like to ask the authors to increase the level of detail in their methodology section with the goal of the work being fully reproducible. Reviewer 2 has provided some excellent feedback to help guide your revision of the presentation of the methods, in particular.
I look forward to your revision!
Sincerely,
Kristan Reed
Animal Research Scientist
USDA-ARS
Reviewed by anonymous reviewer 1, 13 Sep 2024
This is a well-written paper that showcases a dairy goat behavior ML classification model. The dataset was limited both in the number of animals and behaviors observed, but a strong pipeline for preprocessing accelerometer data and extracting features was presented. The dataset and the presented timeline were made available. Results were well presented with strong discussion. The authors did a good job of addressing the limitations of the study and possible future directions. This is a nice first step to have a standardized feature selection algorithm in place so when more behavior data from more animals is available, the study can be repeated with hopefully better insights on how to predict dairy goat behavior from accelerometer data with ML models.
Title and abstract
Does the title clearly reflect the content of the article? [X] Yes, [ ] No (please explain), [ ] I don't know
Although I believe the title is acceptable as is, maybe it would match the objectives and results obtained by showcasing the pipeline a bit better. Something like "A pipeline for preprocessing and feature extraction of accelerometer data aimed at dairy goat behavior classification using machine learning" would maybe showcase that bit better.
Does the abstract present the main findings of the study? [X ] Yes, [ ] No (please explain), [ ] I don’t know
Introduction
Are the research questions/hypotheses/predictions clearly presented? [X ] Yes, [ ] No (please explain), [ ] I don’t know
Does the introduction build on relevant research in the field? [ X] Yes, [ ] No (please explain), [ ] I don’t know
Materials and methods
Are the methods and analyses sufficiently detailed to allow replication by other researchers? [X ] Yes, [ ] No (please explain), [ ] I don’t know
Are the methods and statistical analyses appropriate and well-described? [X ] Yes, [ ] No (please explain), [ ] I don’t know
Results
In the case of negative results, is there a statistical power analysis (or an adequate Bayesian analysis or equivalence testing)? [ ] Yes, [ ] No (please explain), [ ] I don’t know
Are the results described and interpreted correctly? [X ] Yes, [ ] No (please explain), [ ] I don’t know
Discussion
Have the authors appropriately emphasized the strengths and limitations of their study/theory/methods/argument? [X] Yes, [ ] No (please explain), [ ] I don’t know
Are the conclusions adequately supported by the results (without overstating the implications of the findings)? [X ] Yes, [ ] No (please explain), [ ] I don’t know