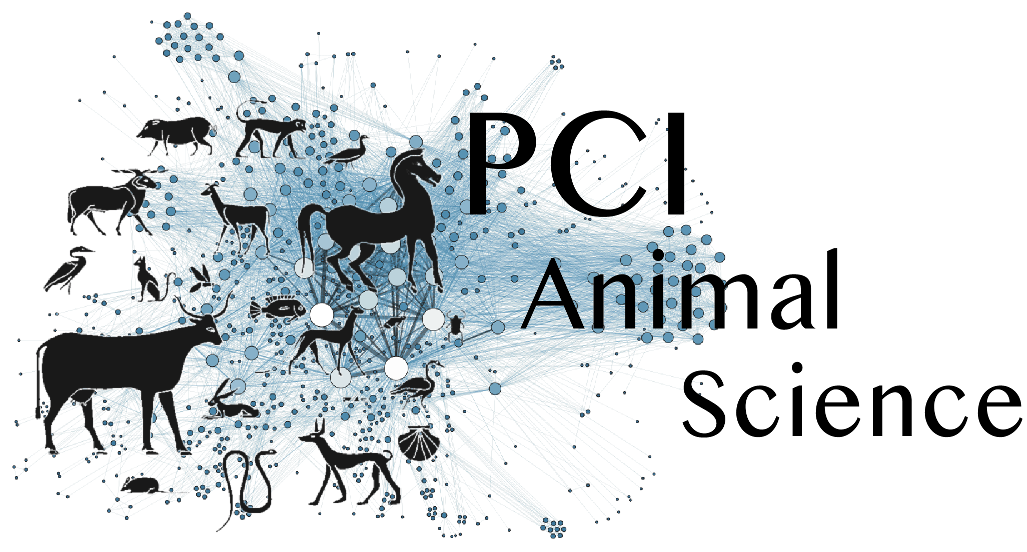
Understanding the mechanisms behind natural bioactive compounds that can potentially reduce methane production in anaerobic conditions. A case study of Asparagopsis taxiformis
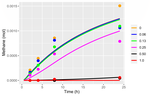
Modelling the impact of the macroalgae Asparagopsis taxiformis on rumen microbial fermentation and methane production
Abstract
Recommendation: posted 12 April 2021, validated 16 April 2021
Tedeschi, L. (2021) Understanding the mechanisms behind natural bioactive compounds that can potentially reduce methane production in anaerobic conditions. A case study of Asparagopsis taxiformis. Peer Community in Animal Science, 100006. https://doi.org/10.24072/pci.animsci.100006
Recommendation
Naturally occurring compounds that can reduce methane production in anaerobic conditions have been studied for quite some time as feasible approaches to mitigate methane production in ruminant animals, especially those of commercial importance. Asparagopsis taxiformis (red algae) and Dictyota bartayresii (brown algae) are effective inhibitors of methane synthesis under in vitro anaerobic fermentation systems (Machado et al., 2014) likely because of their high concentration of secondary metabolites that are toxic to the typical rumen microbiota, including protozoa. In addition to phytoplankton (Palmer and Reason, 2009), Asparagopsis contains a high concentration of haloform compounds (e.g., bromoform, chloroform) while Dictyota has a high concentration of isoprenoid terpenes. Despite the economic and biological impact of diverse phytochemicals on reducing methane production in ruminant animals (Tedeschi et al., 2021), haloform compounds’ environmental impact and safety, in particular, are still unclear. In the present study, Munõz-Tamayo and collaborators (2021) listed relevant literature about the impact of A. taxiformis on ruminal methane production.
Concurrent to the understanding of mechanisms and biology behind the reduction of ruminal methane, mathematical models can lead the way to enhance the effectiveness of feeding A. taxiformis under commercial applications. Thus, in the present study, Munõz-Tamayo and collaborators (2021) sought to develop a mathematical model to understand the rumen fermentation changes in vitro experimentation containing extract of A. taxiformis by adapting a previously documented model by Muñoz-Tamayo et al. (2016).
Modeling development, calibration, and evaluation steps should be independent of each other, requiring complete, distinct, and separate databases (Tedeschi, 2006). However, in rare circumstances where such requirements cannot be met because data availability is scarce, the cross-validation technique, when possible, should be considered to assess data dispersion’s effects on model adequacy. In other situations, clear reasoning for failing to do so must be addressed in the paper. In the present paper, Munõz-Tamayo and collaborators (2021) explained the limitations in their modeling efforts were primarily due to the lack of ideal data: “experiments with simultaneous dynamic data of bromoform, volatile fatty acids, hydrogen, and methane.” Regardless of the availability of ideal data, improvements in the conceptual model are warranted to include amino acids and branched-chain fatty acids fermentation dynamics in the rumen and the fluctuations in ruminal pH.
References
Machado L, Magnusson M, Paul NA, Nys R de, Tomkins N (2014) Effects of Marine and Freshwater Macroalgae on In Vitro Total Gas and Methane Production. PLOS ONE, 9, e85289. https://doi.org/10.1371/journal.pone.0085289
Muñoz-Tamayo R, Chagas JC, Ramin M, Krizsan SJ (2021) Modelling the impact of the macroalgae Asparagopsis taxiformis on rumen microbial fermentation and methane production. bioRxiv, 2020.11.09.374330, ver. 4 peer-reviewed and recommended by PCI Animal Science. https://doi.org/10.1101/2020.11.09.374330
Muñoz-Tamayo R, Giger-Reverdin S, Sauvant D (2016) Mechanistic modelling of in vitro fermentation and methane production by rumen microbiota. Animal Feed Science and Technology, 220, 1–21. https://doi.org/10.1016/j.anifeedsci.2016.07.005
Palmer CJ, Reason CJ (2009) Relationships of surface bromoform concentrations with mixed layer depth and salinity in the tropical oceans. Global Biogeochemical Cycles, 23. https://doi.org/10.1029/2008GB003338
Tedeschi LO (2006) Assessment of the adequacy of mathematical models. Agricultural Systems, 89, 225–247. https://doi.org/10.1016/j.agsy.2005.11.004
Tedeschi LO, Muir JP, Naumann HD, Norris AB, Ramírez-Restrepo CA, Mertens-Talcott SU (2021) Nutritional Aspects of Ecologically Relevant Phytochemicals in Ruminant Production. Frontiers in Veterinary Science, 8. https://doi.org/10.3389/fvets.2021.628445
The recommender in charge of the evaluation of the article and the reviewers declared that they have no conflict of interest (as defined in the code of conduct of PCI) with the authors or with the content of the article. The authors declared that they comply with the PCI rule of having no financial conflicts of interest in relation to the content of the article.
Evaluation round #2
DOI or URL of the preprint: https://doi.org/10.1101/2020.11.09.374330
Version of the preprint: v1
Author's Reply, 12 Apr 2021
Dear Dr. Luis Tedeschi,
Please find below our response to the comments of reviewers.
Review by anonymous reviewer, 2021-03-04 23:45
The authors did not address my concerns. They still use a single study to calibrate their model--or, put more exactly, recalibrate one of their previous models. They still use the same data to calibrate and evaluate the model. The authors made some minor changes to wording, but otherwise their work is the same. My opinion remains that it is too preliminary.
Response: we have recognized the limitation of our study and the need of external data for model validation. While one of the objectives of modelling is to represent experimental data, mathematical modelling provides a tool for knowledge integration, hypothesis testing and for gaining insight on questions that are difficult to elucidate by experimentation. For example, in previous work, the first author exploited the capability of mathematical modelling to identify relevant mechanisms shaping the dynamics of human colonic fermentation even if experimental data were not available (Muñoz-Tamayo et al., 2010). Our work on rumen microbial fermentation deals with the modelling of a complex biological system where it is typical of a situation where the experimental data are very limited and where various and heterogeneous sources of information must be used in combination to get a usable model. What if questions are, for example, as many opportunities of falsifying or improving the model, based on knowledge that does not necessarily take the form of quantitative data. Accordingly, we disagree with the opinion of the reviewer of restricting the application of models to represent experimental data. We believe our work contributes in enhancing the understanding of the dynamics of rumen microbial fermentation.
Review by Henk van Lingen, 2021-03-25 22:40
I appreciate many revisions the authors incorporated, which have clearly improved the quality of the manuscript. However, I still think that the limitations of the present modeling exercise should be more solidly assessed, or at least not be obscured. The authors included a Table with model evaluation statistics about which they now say themselves the data is biased. This point of concern was also raised by one of the other reviewers. I still recommend removing these statistics/Table.
Response: many thanks for your valuable evaluation of our article. With respect to model assessment, we do not think the assessment of our model has been “obscured”. Table 2 shows standard statistical indicators that are widely used in calibration situations such as the one of our article. We are transparent in recognizing that external data is needed for further model validation. However, we do not agree with the suggestion of the reviewer of removing the Table 2. The statistical indicators are here meaningful. For example, if the determination coefficients were very low, we could infer already that the model structure is not adequate for representing our system of study.
I disagree that a global sensitivity analysis will be of great relevance when new data becomes available. A global sensitivity analysis will be relevant, too, when no new data becomes available. It is simply a matter of sampling parameter values and evaluating the relationship with model output. It relates variation in outputs such as methane emissions to uncertainty in parameter values, which is not easily out of the scope of the paper.
Response: the reviewer is right. We were not clear in formulating our response. What we meant is that in this work we did not have the objective of performing global sensitivity analysis. Of course, sensitivity analysis is a useful tool. However, global sensitivity analysis is a domain per se (Saltelli et al., 2000). Rigorous sensitivity analysis is often performed in dedicated articles (Trucchia and Frunzo, 2021). We think that a rigorous sensitivity analysis should be addressed in future work.
The authors have adopted a suggestion by another reviewer to include methane in the title. However, it is then inconsistent that methane in response to A. taxiformis supplementation is not (graphically) shown in the paper as done for hydrogen.
Response: Many thanks for this remark. The figure of methane is now included (Figure 5).
Specific minor comments
Line 250: Not sure if distribution is the right word here as there is no uncertainty estimate (i.e. standard deviation or variance). Would partitioning not be more appropriate?
Response: distribution should be read here as allocation
Why not using the word allocation then?
Response: the word distribution was changed by allocation
“bars” still appears for the x-axis labels in Fig 5.
Response: the correction was done
It seems like the authors had Fig 2 in mind when responding. In Fig 3, an intercept value is shown for the propionate pred vs obs plot, but appears missing for the other 4 plots. Please show this for all 5 plots or explain why the intercept value should only be shown for propionate.
Response: an explanation was added in the legend of the Figure 3. Only the intercept of the curve of propionate was different from zero at 5% significance level.
All typos were corrected
References
Muñoz-Tamayo, R., Laroche, B., Walter, E., Doré, J., and Leclerc, M. (2010) Mathematical modelling of carbohydrate degradation by human colonic microbiota. J Theor Biol 266: 189–201.
Saltelli, A., Chan, K., and Scott, E.M. (2000) Sensitivity analysis, Wiley Series in Probaility and Statistics. John Wiley & Songs, Chichester.
Trucchia, A. and Frunzo, L. (2021) Surrogate based Global Sensitivity Analysis of ADM1-based Anaerobic Digestion Model. J Environ Manage 282: 111456.
Decision by Luis Tedeschi
, posted 04 Apr 2021
Dear authors,
I believe you addressed most of the previous comments and recommendations.
Before a final recommendation by the PCI Anim Sci can be made, for this round of revisions, I invite you to specifically address the comment about the biased evaluation of your model (specifically Table 2) and minor editorial suggestions.
Thanks.
Reviewed by Henk van Lingen, 25 Mar 2021
Reviewed by anonymous reviewer 1, 04 Mar 2021
The authors did not address my concerns. They still use a single study to calibrate their model--or, put more exactly, recalibrate one of their previous models. They still use the same data to calibrate and evaluate the model. The authors made some minor changes to wording, but otherwise their work is the same. My opinion remains that it is too preliminary.
https://doi.org/10.24072/pci.animsci.100047.rev22Evaluation round #1
DOI or URL of the preprint: https://doi.org/10.1101/2020.11.09.374330
Author's Reply, 12 Feb 2021
Dear Dr Luis Tedeschi,
We would like to thank the reviewers for their critical and constructive evaluation of our manuscript that helped to improve our paper
Please find enclosed our reply letter and tacked manuscript
Best regards
Rafael Muñoz-Tamayo
Decision by Luis Tedeschi
, posted 15 Jan 2021
Dear authors,
Four reviewers have provided comments and suggestions on various aspects of your study. Before a final recommendation by the PCI Anim Sci can be made, I invite you to revise your manuscript based on reviewers' feedback.
Please, read carefully the information provided for authors at https://animsci.peercommunityin.org/about/help_generic#For%20authors.
Thanks.
Reviewed by anonymous reviewer 1, 21 Dec 2020
Muñoz-Tamayo et al. developed a mathematical model to explain how Asparagopsis taxiformis inhibits methane formation by rumen microbes in vitro. Previous work has found A. taxiformis inhibits methane formation by up to 80% or more. A mathematical model would be important for understanding at a mechanistic level how this inhibition occurs.
The model developed here could have been valuable, but unfortunately it is too preliminary. The model was calibrated with data from a single study (Chagas et al. 2019) (see L40-1). Further, it was evaluated with the same data as for calibration (see Fig. 3-4 and Table 2). It is not clear why the authors limited themselves to these data, given the number of in vitro and in vivo studies in the literature. To make the conclusions generalizable, more than one study needs to be used. Further, data for calibration and evaluation need to be independent.
https://doi.org/10.24072/pci.animsci.100047.rev11Reviewed by Alberto Atzori, 15 Jan 2021
This paper focus on the adaptation of a dynamic mechanistic model of ruminal fermentation to estimate in vitro effects of macroalgae (i.e. Asparagopsis Taxiformis) rich in natural bioactive compounds as rumen modifier to reduce methane emissions.
The paper is original and focus on two relevant topics for ruminant nutrition: i) optimization of rumen fermentations to mitigate enteric methane emissions and ii) modeling rumen fermentations to improve understanding of fermentation dynamics and to predict fermentation outputs.
The paper can be considered of high quality, is clearly written, detailed and concise in terms of motivation and background, presentation of methodological details, and main findings.
The work is well planned and has strong methodological roots, being based on a published model already evaluated by scientific literature, which was in this work adapted and tested with data from a published experiment on the use of A. Taxiformis in vitro. The presented rumen model has high degree of innovation, since approaches the complex ruminal environment with high level of simplification. The core model assumption is the microbial group aggregation in respect to the use of glucose, aminoacid and Hydrogen influencing VFA and methane production, which were stoichiometrically modeled. Simplified model structure and assumptions do not limit excellent performance in VFA and methane predictions from in vitro fermentations.
Model documentation is complete and exhaustive: model adaptations, in respect to original model versions, are reported in methods with detailed equations, and providing useful links to scripts, model codes and data for readers and users. Model assumptions are explicit and motivated. Origin of model parameters is clearly detailed and motivated (from calibration, literature or from experimental trial of Chagas et al., 2019).
The paper mainly focus on modeling but also biological aspects related with use and effects of A. Taxiformis (and presumably bromoform) as rumen modifier are deeply and concisely discussed referring to broad literature.
Data used to adapt and test the model proceed from a published paper on dose-response in vitro evaluation for the effect of the A. Taxiformis, Chagas et al., 2019. The reference paper is also clearly written and detailed in terms of methods and results. It represented a good basis for model testing and evaluation. The topic focused by Chagas et al., 2019 is highly relevant and the use of A. Taxiformis, and its related bio-active compounds seems highly promising for methane mitigation.
.
Few comments are below highlighted following the sections of paper structure:
Background is mainly focused on biological effects of the Macroalgae and bromoform on ruminal environment explaining biological basis and implication of its use with proper quotes. Lines 25 and 26 might be integrated expliciting if negative long term effects can impair the algae use on animal diets (see also line 348).
A very little part of introduction is dedicated to importance of rumen modeling, which seems a not secondary focus of the paper, especially for microbiological based models. it could represent a frontier in ruminant nutrition. In this sense the paper objective might be integrated with the generic objective of enriching rumen modeling, adaptation and testing and especially accounting for effects of bioactive compounds (as further stated by the authors in line 272) on fermentation complexity.
Methods are well detailed, the model adaptation is justified with proper assumptions and references and documented for high degree of replicability. The model well resumes the rumen complexity. Statistics for model evaluation are adequate. Line 61 refers to four groups then in parenthesis and in Figure 1 only three groups are presented, it should be clarified.
Results of model predictions and sequent evaluations are noticeably presented with multiple figures, enhancing the satisfactory model performances.
Discussions covered both biological and modeling aspects also proposing future model expansions. Among discussions of hydrogen control, an additional sentence can emphasize effects on energy harvesting. In fact, it is quite debated in literature the fact that methane reduction not always lead to higher energy efficiency use, by microbial groups at rumen level or for the animal, whit not significant difference in production performances. Model limitations are separately and exhaustively discussed by the authors both in terms of used data, model assumption and structure with respective proposals for model improvement, including applications for in vivo conditions.
In summary this paper represents a valuable example of valorization of experimental data for model testing and expansion. From these considerations the paper is highly recommended both for quality and findings.
Reviewed by anonymous reviewer 2, 07 Dec 2020
This manuscript presents a model evaluating the impact of Asparagopsis taxiformis on rumen fermentation. The manuscript addresses a relevant topic for this journal. Overall, the manuscript is generally well written although the presentation is likely too specialized for many if not most readers. The authors provide a good introduction describing the background and rationale for their research. The materials and methods are written succinctly but leave out some important details that would help the readers more clearly understand and judge their work.
1) For instance, what was the diet of the two Swedish Red cows? Was it the same as used for the incubations? It is not clear.
2) Were the cows cannulated or was the rumen fluid collected via stomach tube or ruminocentesis?
3) What was the buffer used for the incubations and was it of sufficient buffering capacity to maintain the pH during incubation at or near your assumed pH 6.6 value?
4) How were gases, VFA, ammonia measured? Perhaps this info is in the Ramin and Huhtanen (2012) citation but if so please describe it that way.
5) On lines 109-110, did you really calculate numbers of hydrogen-utilizing microbes as mol/L?
6) Figure 1 presents the model as a closed system, is this true for your incubations or was there venting of gases? If truly a closed system I suspect increasing gas pressures would affect gas solubility.
The results are presented and discussed objectively. You do not need to address this but I am curious to know if you had foaming in your incubations which could potentially be an unaccounted electron sink? I am thinking most fermentation balances do not generally get such good agreement between reducing equivalents consumed and produced as presented here. If you buffer was sufficient to maintain pH at or near 6.6 then that is fine, otherwise I would have liked to see pH measurements.
Overall, this is a good piece of work but I think it would be better if the authors make the effort to make it more broadly readable.
https://doi.org/10.24072/pci.animsci.100047.rev13