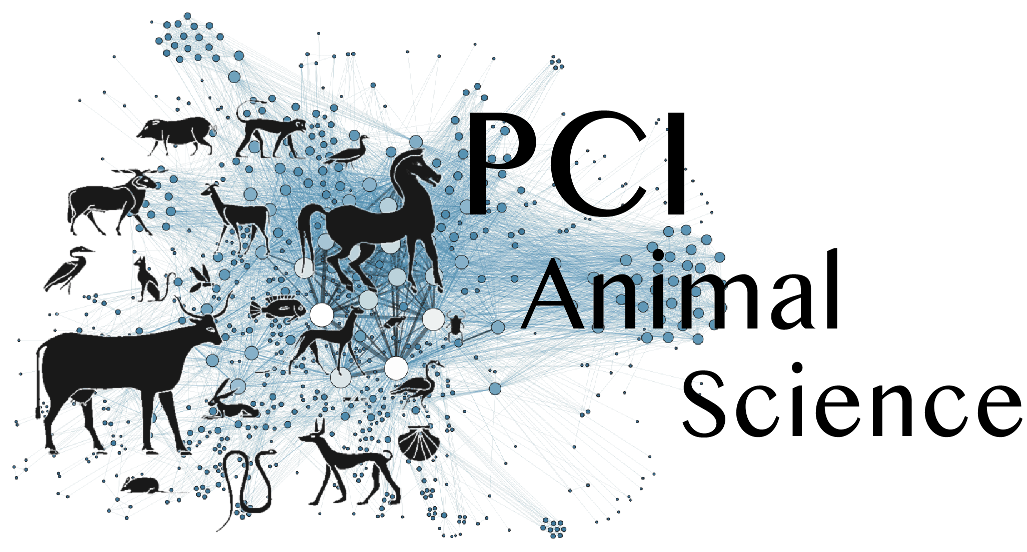
The price of sequencing the livestock genomics
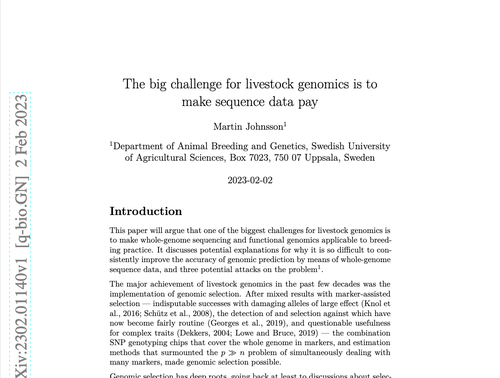
The big challenge for livestock genomics is to make sequence data pay
Abstract
Recommendation: posted 27 July 2023, validated 31 July 2023
Pszczoła, M. (2023) The price of sequencing the livestock genomics. Peer Community in Animal Science, 100192. 10.24072/pci.animsci.100192
Recommendation
Using sequence data in livestock genomics has often been regarded as a solution to revolutionize livestock breeding (Meuwissen & Goddard, 2010). The main expected benefits were to enhance the accuracy of breeding values, achieve better persistence of the accuracy over generations, and enable across populations or breed predictions (Hickey, 2013). Despite the promised benefits, whole-genome sequencing has not yet been implemented in livestock breeding programs, replacing SNP arrays for routine evaluation.
In this work, Johnsson (2023) thoroughly reviewed the literature regarding the implications of whole-genome sequencing and functional genomics for livestock breeding practice. The author discusses the potential applications and reasons for difficulties in their implementation. The author speculates that the main challenge for making using the sequence data profitable is to overcome the problem of the small dimensionality of the genetic data and proposes three potential ways to achieve this goal. The first approach is better modeling of genomic segments, the second inclusion of undetected genetic variation, and the third use of functional genomic information.
The paper presents an original and interesting perspective on the current status of the use of sequence data in livestock breeding programs and perspectives for the future.
References
Hickey,J.M.,2013.Sequencing millions of animals for genomic selection 2.0. Journal of Animal Breeding and Genetics 130:331–332. https://doi.org/10.1111/jbg.12054
Johnsson, M., 2023. The big challenge for livestock genomics is to make sequence data pay. arXiv, 2302.01140, ver. 4 peer-reviewed and recommended by Peer Community in Animal Science. https://doi.org/10.48550/arXiv.2302.01140
Meuwissen, T., Goddard, M.,2010. Accurate prediction of genetic values for complex traits by whole-genome resequencing. Genetics 185:623–631. https://doi.org/10.1534/genetics.110.116590
The recommender in charge of the evaluation of the article and the reviewers declared that they have no conflict of interest (as defined in the code of conduct of PCI) with the authors or with the content of the article. The authors declared that they comply with the PCI rule of having no financial conflicts of interest in relation to the content of the article.
Formas -- A Swedish Research Concil for Sustainable Development
Evaluation round #2
DOI or URL of the preprint: https://doi.org/10.48550/arXiv.2302.01140
Version of the preprint: 3
Author's Reply, 25 Jul 2023
I have corrected the language errors pointed out by Mario Calus.
I've also changed the phrasing about "this information" on page 8, where he pointed out that I had accidentally introduced an ambiguity when revising the passage. Instead it now reads "the additional information from many more genetic variants".
Furthermore, Jang et al. (2022) which I cited in preprint form, has now been published. I updated the citation to Jang et al. (2023) GSE and also the quotation, because the published version says "limited benefits" instead of "limited implications".
Decision by Marcin Pszczoła, posted 23 Jul 2023, validated 24 Jul 2023
The reviewers think that the paper is good for publication. One reviewer suggested small corrections. After the author will consider them I will be happy to recommend this preprint!
Reviewed by anonymous reviewer 1, 21 Jun 2023
This is good enough. No further comments.
Reviewed by Mario Calus, 09 Jul 2023
I think the current version is just fine; it is a pleasure to read and it’s nice to see this overview of the literature and recent developments.
I only have a few minor textual suggestions left that the author may want to consider:
Page 3, 8th line from the bottom: add “to” after “them”
Page 6, middle paragraph: “the they” => “they”
Page 8, 3th line from the top: It is not clear what “that information” refers to, as in the previous sentence you mentioned both sampling more animals and increasing marker density. So perhaps you are referring to both? Please clarify.
Page 13, 6th line from the top: consider to rewrite “and part that” to “and another part is that”
Page 18, 12th line from the top: “the idea to use” => “the idea is to use”
Evaluation round #1
DOI or URL of the preprint: https://doi.org/10.48550/arXiv.2302.01140
Version of the preprint: 2
Author's Reply, 06 Jun 2023
I’m grateful to the reviewers for taking the manuscript seriously and providing many good suggestions. In response to these comments, I have:
• Added a paragraph about the difference between breeding value prediction and resolution of causative variants (in the “Mental models” section).
• Tried to clarify the potential problems with imputation. This is the one point where it seems the reviews might, with Mario Calus asking for empirical evidence that imputation might miss recombination breakpoints, and the second reviewer calling imputation “an extraordinary weakness” that undermines the whole enterprise of genomic prediction with sequence data. I hope this will improve the text regardless of one’s stance on imputation.
• Added a paragraph about the problems of representing non-SNPs from sequence data compared to biallelic SNPs (in the “Better modelling of genomic segments” section), and a paragraph emphasising the different mutation and recombination properties of structural variants compared to SNPs (in the “Inclusion of undetected genetic variation” section).
• Added a paragraph about computational costs to the “Pay for what?” section.
• Made several other smaller edits to clarify arguments about limited dimensionality of genomic information, pre-selection and variable selection, functional genomics, uses for other omics etc.
• Corrected a number of language errors and unclear phrasings pointed out by the reviewers, as well as a few that I spotted while re-reading the manuscript.
Please see point-by-point responses below. I’ve attached a version of the manuscript with tracked changes and line numbers.
Revision needed.
Both reviewers found the paper interesting and commented on improving the manuscript in some aspects. I would like therefore to invite the author to consider the reviewers' opinions and adjust the manuscript accordingly or discuss the raised points.
Reviews
Reviewed by Mario Calus, 12 Mar 2023 13:15
This paper discusses on how to potentially capitalize on the expected benefit of whole genome sequence data for livestock breeding. It is nice to see all ideas and results being presented together. Overall, the paper is well-written and easy to follow. Below I have a few comments that may be useful to improve the paper further.
Main comments:
Line 271-274: “Because farm animal populations are small, at some point, it does not matter
much how many more individuals or genetic variants we sample, because they contain more
of the same information. It seems to me that this limit was reached earlier than geneticists
expected.” I’m not completely sure what your point here is. It is clear that increases in prediction accuracy are subject to diminishing returns. Within a given window of e.g. training population size, the accuracy may appear to reach an asymptote. If the range of training population sizes is increased ten-fold, further increases are observed, and again the accuracy may appear to reach an asymptote.
Response: Yes, this passage was not particularly well written. I mixed two issues together and ended up with something incorrect. The relevant point here is that for a given training population, adding more markers will at some point not add any new information. I also mixed in the observation that, for a given marker density, there is a limit to the number of animals that it is useful to genotype, but that is somewhat beside the point. I’ve rephrased this passage.
Lines 318-320: “Regardless, the lower-density marker panel may not be able to capture all the breakpoints between genomic segments, i.e., recombination event that have happened in the population.” Can you provide any reference to support this, preferably one that has actually demonstrated this?
Response: I was trying to say something simpler, and in some sense self-evident, namely that the information that can be recovered from a lower density marker is limited by marker density. We can know that a switch between haplotypes happened between marker A and B, but not where in that interval it happened. The average marker distance from a medium-density chip in a mammal would be 50-60 kbp, and not all markers will necessarily be informative.
I don’t know of studies that specifically tested this question. When evaluating sequence imputation, researchers mostly seem to study genotype concordance or correlation with true genotype, not location of recombination breakpoints – or when evaluating phasing, counting switch errors. Ferdosi et al. (2014) show a nice visual example of how different phasing methods infer recombination breakpoints on the same data, but those methods are not representative of sequence imputation. In Johnsson, Whalen et al. (2021), we ran a simulation to test our method for estimating autosomal crossover counts based on multilocus peeling, a similar approach as has been used for sequence imputation. While the performance was acceptable, there was also a lot of uncertainty about the number of recombination events. Recombination estimation is not an easy problem, and it stands to reason that locating them is as well.
I have rephrased this passage to put less emphasis on the resolution of segment breakpoints.
Lines 344-345: “or by some Bayesian mixture model that does variable selection based on data” In my experience the Bayesian models are not able to properly select the relevant variants from millions of variants, and subsequently give them the appropriate variances. So, please provide some support (references) for this statement.
Response: In my experience neither – I really only mentioned variable selection here to acknowledge that pre-filtering and variable selection are the two options that have been used before. The purpose of this paragraph is to introduce the idea of improved representations of genomic segments as an improvement on upon these methods. I’ve edited this passage to be less specific; there is no need to highlight Bayesian mixture models in particular, really, only the idea of models that do variable selection as opposed to pre-filtering.
As you write, the results are mixed. When discussing the state of the art for genomic prediction with whole-genome sequence, I’ve cited both positive and negative examples, e.g. Calus et al. (2016) and Meuwissen et al. (2021) – in the latter, variable selection seems to perform comparable to pre-selection, i.e., improve accuracy a little in some scenarios.
Lines 356-358: “Proposals to better deal with this includes defining windows based on recombination hotspots (Oppong et al., 2022) or haplotype block methods that create overlapping segments (Pook et al., 2019).” There are some examples that are perhaps making a better attempt at this. For instance: Beissinger, T., Rosa, G., Kaeppler, S., Gianola, D., de Leon, N., 2015. Defining window-boundaries for genomic analyses using smoothing spline techniques. Genetics Selection Evolution 47, 30. doi:10.1186/s12711-015-0105-9.
Response: I’ve added a citation to this section about haplotype clustering methods. However, Beissinger et al. (2015) deals defining the windows in genome-wide population genetic analyses (their example is an Fst scan based on pooled sequence data) based on fitting splines to the summary statistics themselves. It explicitly does not use linkage disequilibrium or haplotype blocking – that information is hard to get from pooled sequence, as discussed at the end of their Discussion. I don’t see how it is applicable here. They hint at the idea of using splines applied to summary statistics as a way to infer LD structure, but I could not find related work by the authors following up on this.
Line 426: “Unfortunately, a discouraging number of damaging variants and causative variants” Is this number discouraging high or low? Please add to clarify.
Response: I changed this to “discouragingly high” with the additional qualification that this is discouraging if we hoped that variant called from short-read sequencing would include causative variants.
Line 579: “did not find a benefit to weighting” Weights can be considered to be prior information. Considering Bayes Theorem, this benefit may be quite depending on the size of the training data, and is expected to decrease with increasing training population size.
Response: Yes, the sample size of the study is relevant here. Also, I failed to point out in the text that we’re kind of comparing apples to pears here. The analysis from Fragomeni et al. that suggests that weights are important used the true simulated effects for weighting, whereas Jang et al. estimated their weights from marker effects in GWAS, and they were likely not that accurate. When Fragomeni et al. used weights derived from GWAS, they also only led to a minor improvement in accuracy. I’ve edited the passage to try to make this clearer.
Lines 667-669: “a microbiome sample or an epigenomic sample may also contain useful information about the environment” In addition, these other omics could be useful for instance to predict phenotypes of traits related to them (e.g. feed efficiency, feed digestibility).
Response: Good point. I have rephrased this passage to include phenotype prediction.
Detailed comments:
Line 22: should “of” be added here? E.g. “the combination of SNP genotyping chips”
Line 28: “the identifying” => “identifying”
Line 42: “gist” I had to look up this word; you may want to consider to use a different word instead.
Line 117: “a bigger breeds” should be “a bigger breed” or “bigger breeds”
Line 147: “process pre-selection” => “process of pre-selection”
Lines 147-148: “a genome-wide association studies” => “genome-wide association studies” or “a genome-wide association study”
Line 253: “relatively well even a small amount” I think a word is missing here. Perhaps: “relatively well even with a small amount”
Line 263: Should “and to do” be “and how do” ?
Lines 451-452: “that structural variants can the genotyping of neighbouring variants, by changing flaking sequence” There is something wrong in this phrase. Please check and rephrase. In any case: “flaking” => “flanking”
Line 463: it is not clear what “these results” refers to. Please make that explicit.
Line 500: should “enhances” be “enhancers” ?
Line 558: “assumption” => “assumptions”
Line 565: “have an edge over observational methods open chromatin analyses” something appears to be missing here. Please check and rephrase.
Response: Thank you! I have edited all these passages to correct the errors and improve the unclear phrasings.
Reviewed by anonymous reviewer, 26 Mar 2023 18:01
PCI Anim Sci #192
This opinion paper is fun to read and informative. I think however that they could be improved by the author making a few relevant points (probably a couple of paragraphs at the introduction or the end) that I propose (feel free to disagree):
- the paper perspirates the feeling of disappointment because sequence is not more accurate than SNP chips. However, 100 years ago it was well verified that it was enough to measure mil yield once a month and not every day
Response: If the reviewer is saying that one may also have a positive take on the issue, the point is well taken. Viewed from a different perspective, it is great news that SNP chips are doing so well compared to the much more expensive and cumbersome sequencing. I have added a passage to this effect.
- predicting region effects and BV are different things. In my view scientists think that the former implies the latter, but the regression+prediction framework that we all use does not imply causation - assigning effects to regions does not imply that they're true..
Response: I agree. I believe it is a mistake to think of GWAS-results as straightforward reflections of causative variants, when they are patterns that can result from many different genetic processes. I struggled a little to find the proper place to fit this argument into the manuscript; I’ve added a paragraph to the “Mental models of genomic selection”, near the end where I discuss the ephemeral nature of marker effects.
- the costs and organisation involved are not negligible. Storage increases linearly (from 50K to say 20M the increase is a factor of 1000) and computation increases, at best, quadratically. So if a SNPBLUP with 50K SNPs and 1M animals take say 3 hours, a SequenceBLUP will take a few days. Pre-selection is not necessarily cheaper because it will be linear and will (likely) need to be updated
Response: This is a good point that I had mostly neglected. I’ve added some discussion about costs to the “Pay for what?” section.
- is functional genomic sequence data "sequence data"? I would argue that these are extra measures like CT scan of pigs or flow in a milking machine.
Response: When is genomic data not genomic? My take is that it makes sense to think of functional genomics as sequence data when its genomic coordinates are used to analyse genetic variants. If e.g., RNA-seq data is used for eQTL mapping or prediction of an animal-level trait, then I agree with the reviewer, that it is more like a high-dimensional phenotype, that can be lumped in with “other omics”. If it is used for fine-mapping of causative variants or for variable selection/pre-selection in a genomic prediction context, I would think of it as “sequencing” and “genomics”. I think this is a good point to think more about, but I’ve not made any edit based on it.
Also I have some other, minor points (except maybe the last one, which I see as more relevant)
15 here you should mention costs
Response: Good point. I’ve added a sentence about the substantial costs of sequencing to the first paragraph.
23 now there's no more a problem of p>>n in most populations. But SNPs being random effects makes complete sense in a geneticist view (at least this geneticist).
Response: I agree. I’ve added this to the paragraph.
39 the point that SNP is well behaved is really important. To me one of the problems of sequence analyses is that either you treat as SNP (which makes no biological sense) or as haplotypes, etc (and then there's lots of compromises). Author emphasizes this well. In other words, sequence data does not lead itself to a manner of analysing, contrary to SNP data.
Response: While the text returns to this point later in more detail, I added a passage to this paragraph to emphasise that sequence data lacks these convenient properties.
75 DMRT3 in horses (doi:10.1038/nature11399) is a gene whose causal segregating mutation was in the initial version of the equine chip. Quite astonishing.
Response: That is astonishing! I couldn’t identify a great reference for the SNP chip and variant in question, otherwise this would have made a very nice anecdote to add here.
76 a problem here is that we don't have a real model for "causative variant", but definitely in many genes it is *not* SNPs. IT may be a series of them in a few kbs or even worse, insertions, deletions, etc. Perhaps this is worth mentioning.
Response: This point was touched upon in the “Inclusion of undetected genetic variation” section, but maybe it deserved more reasoning. I’ve added a paragraph to go deeper into what might be different about structural variants and other non-SNP causative variants.
110 but the point that everyone preferred to ignore is that even if genes are better tagged using sequence data, later prediction is for entire animals, whose genomes *are* in LD. So may linear combinations of estimates yield the same accuracy.
Response: I think this is an important point, and I’ve tried to incorporate it into the new paragraph in the “Mental models” section (see above).
143 a notable example in which including a large causal gene (SOCS2) did *not* improve accuracy is Oget et al. https://doi.org/10.1186/s12864-019-6068-4 . I have also been told that including the causal mutation of DGAT1 in dairy cattle does not improve accuracy either because surounding SNPs capture the effect. After thinking, this is kind of obvious because both genes (DGAT1 and SOCS2) have been found *because* there were SNPs tagging them. So perhaps sequence data would be interesting if it could tag genes not tagged by SNP markers? is this worth mentioning?
Response: I think this is a useful point – I feel like the section on including undetected genetic variation kind of circles a similar argument, and I’ve edited it to emphasise that the point is to get at variation that isn’t already well tagged by SNP chips.
207 see my comment in 110
Response: I’ve tried to incorporate it into the new paragraph in the “Mental models” section, a few paragraphs down from this one.
244 if I remember correctly, Beagle (maybe it's another program) has a notion of "fuzzy" haplotypes and this would be a way out of these arbitrary choices.
Response: I’ve added a citation to Beagle’s haplotype clustering.
275 but strangely enough, SNP effects hold during at least 2 generations at least in dairy cattle. My personal opinion is that this is because they have been "trained" with records from ~5 generations.
Response: I didn’t make any edit based on this comment, but I agree that might be a story here about the “age/genetic distance composition” of the training set and persistence of accuracy.
310 this is an extraordinay weakness and in my opinion, even if sequence data per se were more accurate (which may or may not) the fact of imputing will likely make it fail (the author is a bit ambigous on this at the end of the manuscript). And if we sequence all animals then we have enormous costs of storing and bioinformatics.
Response: This seems to be the one point where the reviewers (maybe) disagree about how serious the imputation issues are. In response to the other reviewer, I’ve rephrased the passage about weaknesses of imputation. I also rephrased a sentence later about the implications of simulations with perfect data, which also struggle to gain any benefit from sequence data. They do not, as I previously phrased it, necessarily show that “imputation isn’t the main problem”, just that even if imputation was not a problem, there would be other limitations was well.
346 fully agree, see my comment in 244
Response: Thank you! I’ve tried to add some detail about representation issues for non-SNPs, and a citation to Browning & Browning about haplotype clustering.
378 see my point in 76
Response: As mentioned above, I’ve added a paragraph to go deeper into what might be different about structural variants and other non-SNP causative variants later in this section.
499 - 513 after casual reading of these papers, I have the feeling that the improvement observed is largely due to cherry-picking of the best options tried among many, and of small data sets. A bit like Bayesian regressions seemed to perform better than GBLUP but with large data sets they are equally accurate.
Response: That may very well be. The testing sets used are generally quite small, some of the studies don’t test prediction accuracy but only variance explained within sample, and I think it’s fair to say that a full-scale test of this kind of methodology is lacking. I’ve softened the claims in this section.
548 this would imply doing as many assays as traits? is this really "sequence data"?
Response: I see where you’re coming from. Yes, I think any realistic attempt at variant prioritisation with functional genomics would need to target many tissues and cell types, likely at least one per major trait complex – say, major cell types in the udder, sampled during different parts of lactation; different skeletal muscles sampled during a developmental time course etc. One might even need to perform an eQTL study for each of them. I think the hope is that such genomic datasets will be universal enough that they don’t have to be repeated for each line/breed and over the generations, but can serve as a form of “genome annotation” with longer shelf life than typical training data. To the extent that they are used for variant annotation, I think they can still be thought of as “genomics” and “sequence data”. I’ve also changed the section title to “Use of functional genomic information”, because that better encapsulates the point of the section.
592 I'm not convinced at all. Yengo says "12,111 independent SNPs that are significantly associated with height account for nearly all of the common SNP-based heritability. These SNPs are clustered within 7,209 non-overlapping genomic segments with a mean size of around 90 kb, covering about 21% of the genome.". That means that on average there are 7209/26 = 277 regions per chromosome which for me it's a lot anyway. Imagine that we describe each of this regions with 4 SNP markers: we have ~28K markers. Add the markers needed for other regions associated to other traits. Very quickly we're back on the pan-genome 50K chip.
Response: Yes, it seems likely that any such panel would grow beyond the size of a normal 50k chip, especially with regions for all the relevant traits. I’ve rephrased the latter part of this section to include this point. I also deleted the point about the omnigenic model, because upon thinking about it more, this is a bit beside the point.
Decision by Marcin Pszczoła, posted 31 Mar 2023, validated 01 Apr 2023
Both reviewers found the paper interesting and commented on improving the manuscript in some aspects. I would like therefore to invite the author to consider the reviewers' opinions and adjust the manuscript accordingly or discuss the raised points.
Reviewed by Mario Calus, 12 Mar 2023
This paper discusses on how to potentially capitalize on the expected benefit of whole genome sequence data for livestock breeding. It is nice to see all ideas and results being presented together. Overall, the paper is well-written and easy to follow. Below I have a few comments that may be useful to improve the paper further.
Main comments:
Line 271-274: “Because farm animal populations are small, at some point, it does not matter
much how many more individuals or genetic variants we sample, because they contain more
of the same information. It seems to me that this limit was reached earlier than geneticists
expected.” I’m not completely sure what your point here is. It is clear that increases in prediction accuracy are subject to diminishing returns. Within a given window of e.g. training population size, the accuracy may appear to reach an asymptote. If the range of training population sizes is increased ten-fold, further increases are observed, and again the accuracy may appear to reach an asymptote.
Lines 318-320: “Regardless, the lower-density marker panel may not be able to capture all the breakpoints between genomic segments, i.e., recombination event that have happened in the population.” Can you provide any reference to support this, preferably one that has actually demonstrated this?
Lines 344-345: “or by some Bayesian mixture model that does variable selection based on data” In my experience the Bayesian models are not able to properly select the relevant variants from millions of variants, and subsequently give them the appropriate variances. So, please provide some support (references) for this statement.
Lines 356-358: “Proposals to better deal with this includes defining windows based on recombination hotspots (Oppong et al., 2022) or haplotype block methods that create overlapping segments (Pook et al., 2019).” There are some examples that are perhaps making a better attempt at this. For instance: Beissinger, T., Rosa, G., Kaeppler, S., Gianola, D., de Leon, N., 2015. Defining window-boundaries for genomic analyses using smoothing spline techniques. Genetics Selection Evolution 47, 30. doi:10.1186/s12711-015-0105-9.
Line 426: “Unfortunately, a discouraging number of damaging variants and causative variants” Is this number discouraging high or low? Please add to clarify.
Line 579: “did not find a benefit to weighting” Weights can be considered to be prior information. Considering Bayes Theorem, this benefit may be quite depending on the size of the training data, and is expected to decrease with increasing training population size.
Lines 667-669: “a microbiome sample or an epigenomic sample may also contain useful information about the environment” In addition, these other omics could be useful for instance to predict phenotypes of traits related to them (e.g. feed efficiency, feed digestibility).
Detailed comments:
Line 22: should “of” be added here? E.g. “the combination of SNP genotyping chips”
Line 28: “the identifying” => “identifying”
Line 42: “gist” I had to look up this word; you may want to consider to use a different word instead.
Line 117: “a bigger breeds” should be “a bigger breed” or “bigger breeds”
Line 147: “process pre-selection” => “process of pre-selection”
Lines 147-148: “a genome-wide association studies” => “genome-wide association studies” or “a genome-wide association study”
Line 253: “relatively well even a small amount” I think a word is missing here. Perhaps: “relatively well even with a small amount”
Line 263: Should “and to do” be “and how do” ?
Lines 451-452: “that structural variants can the genotyping of neighbouring variants, by changing flaking sequence” There is something wrong in this phrase. Please check and rephrase. In any case: “flaking” => “flanking”
Line 463: it is not clear what “these results” refers to. Please make that explicit.
Line 500: should “enhances” be “enhancers” ?
Line 558: “assumption” => “assumptions”
Line 565: “have an edge over observational methods open chromatin analyses” something appears to be missing here. Please check and rephrase.
Reviewed by anonymous reviewer 1, 26 Mar 2023
PCI Anim Sci #192
This opinion paper is fun to read and informative. I think however that they could be improved by the author making a few relevant points (probably a couple of paragraphs at the introduction or the end) that I propose (feel free to disagree):
- the paper perspirates the feeling of disappointment because sequence is not more accurate than SNP chips. However, 100 years ago it was well verified that it was enough to measure mil yield once a month and not every day
- predicting region effects and BV are different things. In my view scientists think that the former implies the latter, but the regression+prediction framework that we all use does not imply causation - assigning effects to regions does not imply that they're true..
- the costs and organisation involved are not negligible. Storage increases linearly (from 50K to say 20M the increase is a factor of 1000) and computation increases, at best, quadratically. So if a SNPBLUP with 50K SNPs and 1M animals take say 3 hours, a SequenceBLUP will take a few days. Pre-selection is not necessarily cheaper because it will be linear and will (likely) need to be updated
- is functional genomic sequence data "sequence data"? I would argue that these are extra measures like CT scan of pigs or flow in a milking machine.
Also I have some other, minor points (except maybe the last one, which I see as more relevant)
15 here you should mention costs
23 now there's no more a problem of p>>n in most populations. But SNPs being random effects makes complete sense in a geneticist view (at least this geneticist).
39 the point that SNP is well behaved is really important. To me one of the problems of sequence analyses is that either you treat as SNP (which makes no biological sense) or as haplotypes, etc (and then there's lots of compromises). Author emphasizes this well. In other words, sequence data does not lead itself to a manner of analysing, contrary to SNP data.
75 DMRT3 in horses (doi:10.1038/nature11399) is a gene whose causal segregating mutation was in the initial version of the equine chip. Quite astonishing.
76 a problem here is that we don't have a real model for "causative variant", but definitely in many genes it is *not* SNPs. IT may be a series of them in a few kbs or even worse, insertions, deletions, etc. Perhaps this is worth mentioning.
110 but the point that everyone preferred to ignore is that even if genes are better tagged using sequence data, later prediction is for entire animals, whose genomes *are* in LD. So may linear combinations of estimates yield the same accuracy.
143 a notable example in which including a large causal gene (SOCS2) did *not* improve accuracy is Oget et al. https://doi.org/10.1186/s12864-019-6068-4 . I have also been told that including the causal mutation of DGAT1 in dairy cattle does not improve accuracy either because surounding SNPs capture the effect. After thinking, this is kind of obvious because both genes (DGAT1 and SOCS2) have been found *because* there were SNPs tagging them. So perhaps sequence data would be interesting if it could tag genes not tagged by SNP markers? is this worth mentioning?
207 see my comment in 110
244 if I remember correctly, Beagle (maybe it's another program) has a notion of "fuzzy" haplotypes and this would be a way out of these arbitrary choices.
275 but strangely enough, SNP effects hold during at least 2 generations at least in dairy cattle. My personal opinion is that this is because they have been "trained" with records from ~5 generations.
310 this is an extraordinay weakness and in my opinion, even if sequence data per se were more accurate (which may or may not) the fact of imputing will likely make it fail (the author is a bit ambigous on this at the end of the manuscript). And if we sequence all animals then we have enormous costs of storing and bioinformatics.
346 fully agree, see my comment in 244
378 see my point in 76
499 - 513 after casual reading of these papers, I have the feeling that the improvement observed is largely due to cherry-picking of the best options tried among many, and of small data sets. A bit like Bayesian regressions seemed to perform better than GBLUP but with large data sets they are equally accurate.
548 this would imply doing as many assays as traits? is this really "sequence data"?
592 I'm not convinced at all. Yengo says "12,111 independent SNPs that are significantly associated with height account for nearly all of the common SNP-based heritability. These SNPs are clustered within 7,209 non-overlapping genomic segments with a mean size of around 90 kb, covering about 21% of the genome.". That means that on average there are 7209/26 = 277 regions per chromosome which for me it's a lot anyway. Imagine that we describe each of this regions with 4 SNP markers: we have ~28K markers. Add the markers needed for other regions associated to other traits. Very quickly we're back on the pan-genome 50K chip.